People
Publications
Preprints
- Kohlhaas, R., Hommel, J., Weinhardt, F., Class, H., Oladyshkin, S., & Flemisch, B. (2025). Numerical Investigation of Preferential Flow Paths in Enzymatically Induced Calcite Precipitation supported by Bayesian Model Analysis. In arXiv preprint arXiv:2503.17314. https://doi.org/10.48550/arXiv.2503.17314
- Wang, W., Zhang, X., Bezgin, D., Buhendwa, A., Chu, X., & Weigand, B. (2024). JAX-based differentiable fluid dynamics on GPU and end-to-end optimization. In arXiv preprint arXiv:2406.19494. https://doi.org/10.48550/arXiv.2406.19494
Conference papers
- Jaust, A., Weishaupt, K., Mehl, M., & Flemisch, B. (2020). Partitioned coupling schemes for free-flow and porous-media applications with sharp interfaces. In R. Klöfkorn, E. Keilegavlen, F. A. Radu, & J. Fuhrmann (Eds.), Finite Volumes for Complex Applications IX - Methods, Theoretical Aspects, Examples (Vol. 323, pp. 605–613). Springer International Publishing. https://doi.org/10.1007/978-3-030-43651-3_57
(Journal-) Articles
- Kröker, I., Brünnette, T., Wildt, N., Morales Oreamuno, M. F., Kohlhaas, R., Oladyshkin, S., & Nowak, W. (2025). Bayesian3 active learning for regularized multi-resolution arbitrary polynomial chaos using information theory. International Journal for Uncertainty Quantification, 15, Article 3. https://doi.org/10.1615/Int.J.UncertaintyQuantification.2024052675
- Flemisch, B., Nordbotten, J. M., Fernø, M., Juanes, R., Both, J. W., Class, H., Delshad, M., Doster, F., Ennis-King, J., Franc, J., Geiger, S., Gläser, D., Green, C., Gunning, J., Hajibeygi, H., Jackson, S. J., Jammoul, M., Karra, S., Li, J., et al. (2024). The FluidFlower Validation Benchmark Study for the Storage of CO2. Transport in Porous Media, 151, 865–912. https://doi.org/10.1007/s11242-023-01977-7
- Nordbotten, J. M., Ferno, M. A., Flemisch, B., Kovscek, A. R., & Lie, K.-A. (2024). The 11th Society of Petroleum Engineers Comparative Solution Project: Problem Definition. SPE Journal, 29, 2507–2524. https://doi.org/10.2118/218015-PA
- Bauer, R., Ngo, Q. Q., Reina, G., Frey, S., Flemisch, B., Hauser, H., Ertl, T., & Sedlmair, M. (2023). Visual Ensemble Analysis of Fluid Flow in Porous Media Across Simulation Codes and Experiment. Transport in Porous Media, 151. https://doi.org/10.1007/s11242-023-02019-y
- Bürkner, P.-C., Kröker, I., Oladyshkin, S., & Nowak, W. (2023). A fully Bayesian sparse polynomial chaos expansion approach with joint priors on the coefficients and global selection of terms. Journal of Computational Physics, 488. https://doi.org/10.1016/j.jcp.2023.112210
- Kohlhaas, R., Kröker, I., Oladyshkin, S., & Nowak, W. (2023). Gaussian active learning on multi-resolution arbitrary polynomial chaos emulator: concept for bias correction, assessment of surrogate reliability and its application to the carbon dioxide benchmark. Computational Geosciences, 27, 369–389. https://doi.org/10.1007/s10596-023-10199-1
- Kröker, I., Oladyshkin, S., & Rybak, I. (2023). Global sensitivity analysis using multi-resolution polynomial chaos expansion for coupled Stokes-Darcy flow problems. Computational Geosciences, 27, 805–827. https://doi.org/10.1007/s10596-023-10236-z
- Mohammadi, F., Eggenweiler, E., Flemisch, B., Oladyshkin, S., Rybak, I., Schneider, M., & Weishaupt, K. (2023). A surrogate-assisted uncertainty-aware Bayesian validation framework and its application to coupling free flow and porous-medium flow. Computational Geosciences, 27. https://doi.org/10.1007/s10596-023-10228-z
- Mouris, K., Espinoza, E. A., Schwindt, S., Mohammadi, F., Haun, S., Wieprecht, S., & Oladyshkin, S. (2023). Stability criteria for Bayesian calibration of reservoir sedimentation models. Modeling Earth Systems and Environment, 9, 3643–3661. https://doi.org/10.1007/s40808-023-01712-7
- Oladyshkin, S., Praditia, T., Kroeker, I., Mohammadi, F., Nowak, W., & Otte, S. (2023). The deep arbitrary polynomial chaos neural network or how Deep Artificial Neural Networks could benefit from data-driven homogeneous chaos theory. Neural Networks, 166, 85–104. https://doi.org/10.1016/j.neunet.2023.06.036
- Schwindt, S., Medrano, S. C., Mouris, K., Beckers, F., Haun, S., Nowak, W., Wieprecht, S., & Oladyshkin, S. (2023). Bayesian calibration points to misconceptions in three-dimensional hydrodynamic reservoir modeling. Water Resources Research, 59. https://doi.org/10.1029/2022WR033660
- Cheng, K., Lu, Z., Xiao, S., Oladyshkin, S., & Nowak, W. (2022). Mixed covariance function Kriging model for uncertainty quantification. International Journal for Uncertainty Quantification, 12, Article 3. https://doi.org/10.1615/Int.J.UncertaintyQuantification.2021035851
- Kröker, I., & Oladyshkin, S. (2022). Arbitrary multi-resolution multi-wavelet-based polynomial chaos expansion for data-driven uncertainty quantification. Reliability Engineering & System Safety, 222. https://doi.org/10.1016/j.ress.2022.108376
- Berre, I., Boon, W. M., Flemisch, B., Fumagalli, A., Gläser, D., Keilegavlen, E., Scotti, A., Stefansson, I., Tatomir, A., Brenner, K., Burbulla, S., Devloo, P., Duran, O., Favino, M., Hennicker, J., Lee, I.-H., Lipnikov, K., Masson, R., Mosthaf, K., et al. (2021). Verification benchmarks for single-phase flow in three-dimensional fractured porous media. Advances in Water Resources, 147. https://doi.org/10.1016/j.advwatres.2020.103759
- Scheurer, S., Silva, A. S. R., Mohammadi, F., Hommel, J., Oladyshkin, S., Flemisch, B., & Nowak, W. (2021). Surrogate-based Bayesian comparison of computationally expensive models: application to microbially induced calcite precipitation. Computational Geosciences, 25, 1899–1917. https://doi.org/10.1007/s10596-021-10076-9
- Seitz, G., Mohammadi, F., & Class, H. (2021). Thermochemical Heat Storage in a Lab-Scale Indirectly Operated CaO/Ca(OH)2 Reactor-Numerical Modeling and Model Validation through Inverse Parameter Estimation. Applied Sciences, 11. https://doi.org/10.3390/app11020682
- Oladyshkin, S., Mohammadi, F., Kroeker, I., & Nowak, W. (2020). Bayesian3 Active Learning for the Gaussian Process Emulator Using Information Theory. Entropy, 22. https://doi.org/10.3390/e22080890
- Oladyshkin, S., & Nowak, W. (2019). The Connection between Bayesian Inference and Information Theory for Model Selection, Information Gain and Experimental Design. Entropy, 21. https://doi.org/10.3390/e21111081
- Schneider, M., Gläser, D., Flemisch, B., & Helmig, R. (2018). Comparison of finite-volume schemes for diffusion problems. Oil & Gas Science and Technology - Revue d’IFP Energies Nouvelles, 73. https://doi.org/10.2516/ogst/2018064
Published data sets
- Flemisch, B., Nordbotten, J. M., Fernø, M., Lie, K.-A., Kovscek, A., Both, J., Møyner, O., Sandve, T. H., Di, C., Chen, Z., Gasanzade, F., Bauer, S., Green, C., Sayyafzadeh, M., Ghomian, Y., Ruby, N., Hadjisotiriou, G., Voskov, D., Franc, J., et al. (2025). The 11th SPE Comparative Solution Project: Submitted Data [DaRUS]. https://doi.org/10.18419/DARUS-4750
- Kohlhaas, R., Morales Oreamuno, M. F., & Lacheim, A. (2025). BayesValidRox 2.0.0 [DaRUS]. https://doi.org/10.18419/DARUS-4752
- Gläser, D., Seeland, A., Schulze, K., & Burbulla, S. (2023). Verification benchmarks for single-phase flow in three-dimensional fractured porous media: DuMuX source code [DaRUS]. https://doi.org/10.18419/DARUS-3228
- Jaust, A., Weishaupt, K., Flemisch, B., & Schulte, M. (2021). Replication Data for: Partitioned coupling schemes for free-flow and porous-media applications with sharp interfaces [DaRUS]. https://doi.org/10.18419/DARUS-1644
Research
About this Project
Project D03 develops, maintains and applies an open-source Bayesian framework for uncertainty-aware validation of computational models in the fields of fluid mechanics and porous media flow, particularly the ones developed in the three application project areas of the SFB 1313. In addition, the project is responsible for model validation and benchmarking efforts between projects of the SFB.
Results
Funding Period 1
In the first funding period, we developed a framework that relies on probabilistic modelling techniques and incorporates available experimental data using a fully Bayesian approach. A Bayesian perspective on a validation task yields an optimal bias-variance trade-off against the experimental data and provides an integrative metric for model validation that incorporates parameter and conceptual uncertainty of the underlying computational models. The developed framework for stochastic model calibration and validation seeks to quantify post-calibration uncertainty for non-linear models with long run times used in Project Areas A to C. Concerning Project Area A, we initiated an uncertainty-aware validation benchmark for coupling free flow and porous-medium flow with the goal of comparing various coupling conditions at the macro scale against a highly resolved pore-scale reference model. Moreover, we applied the Bayesian validation framework to set up a benchmark for models of flow in fractured porous media that resulted from a successful collaboration between SFB members within Project Area B. Related to Project Area C, we used components of the framework to perform a Bayesian justifiability analysis by which we investigated model similarities for geochemical processes in subsurface reservoirs affected by microbial activity.
Funding Period 2
Our Bayesian validation framework was published at the beginning of the current (second) funding period as the open-source Python package BayesValidRox, and has since undergone multiple releases. Making the framework publicly available, we restructured the core of our Bayesian validation framework to maximize flexibility and modularity, allowing mix and match between various options and easy implementation of new features. The workflow for release v2.0.0 is shown in figure 2. Continuing from the first funding period we extended the capability of the framework by incorporating Gaussian properties into surrogate models, enhancing performance accuracy through Bayesian active learning and considering space and time dependency by using dimensionality reduction techniques.
We successfully applied our Bayesian validation framework in collaboration with other projects within the SFB. In particular, we finalized the comparison of conditions for coupling porous-medium flow to free flow with Projects A02 and A03 that was started in FP 1. Figure 2 shows one of the results of this collaboration, pairwise comparisons between conditions using the distributions of Bayes Factors. We are working on an extension of this collaboration where we investigate the impact of different pore geometries, surface roughness and the coupling of Navier-Stokes to Darcy flow on the generalized interface conditions developed by A03. We collaborated with projects B05 and C04 to extend an existing REV-scale model for enzymatically induced calcium carbonate precipitation (CIEP) by a mobile precursor phase, and investigated uncertain model properties and their relation to the production of preferential flow paths in heterogeneous domains. Additionally, in collaboration with Projects B03, B04 and INF we are conducting a comparison study on the stochastic validation of transport models with irregular and uncertain fracture geometries.
We coordinated the FluidFlower benchmark study, a collaborative effort aimed at validating computational models for geological CO2 storage through experimental data. Nine different research groups (one of them the LH2 with H. Class) from around the world provided forecasts, both individually and collaboratively. The results have been analysed, and a visual ensemble analysis was performed in collaboration with project D01. Building on the FluidFlower benchmark, the 11th Society of Petroleum Engineers Comparative Solution Project (the 11th SPE CSP) has been initiated together with partners from Bergen, SINTEF and Stanford. The benchmark consists of three cases that are purely synthetic with a fully specified mathematical model. For each case 21-34 results from 13-18 participating groups were obtained and are being analysed.
Future Work
Our goal for the third funding period is two-fold, further improving our validation framework, especially with respect to complex and coupled models, and setting up and running benchmarks between projects of the SFB.
Additions to the framework will include implementations of methods for physics-informed and multi-output training for surrogates, especially Bayesian sparse polynomial chaos expansions, as well as methods for sensitivity analysis for coupled models. We will improve out model assessment methodology by introducing a Bayesian Pareto approach for multi-objective validation, and collaborating with projects B04 and D01 for validation and visualization related to complex output data, and with project INF for improving applicability on large numbers of models.
For the third funding period we will set up and conduct two open studies that are both connected to the vision topic. Each study is associated with at least two application project areas and our Bayesian framework will be applied to both. The first scenario will consider evaporation-induced salt precipitation, and include experimental capabilities of projects C05, A06 and Z02, as well as models developed by projects C02, C03 and B02. The second scenario will be on biomineralization and include experiments by projects B05, C04 and Z02, as well as models by B01, C01 and C04. Projects D01, D02 and INF will also be involved in the benchmarks with their respective capabilities.
International Collaboration
Politecnico di Milano, Italy
We will collaborate with Prof. Monica Riva at PoliMI in the scope of sensitivity analysis for coupled models. We expect that the advanced sensitivity analysis will provide deeper insights into coupled nonlinear problems, where the interaction between modelling parameters is highly complex and nontrivial. Prof. Riva will also be the host for a three-month research stay of the project researcher in D03.
University of Bergen, Norway; Massachusetts Institute of Technology, USA; SINTEF, Norway; Stanford University, USA
We collaborated on open benchmark studies for geological carbon storage. This includes the FluidFlower International Benchmark Study and the 11th Society of Petroleum Engineers Comparative Solution Project.
For further information please contact
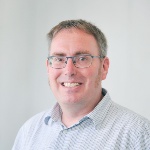
Bernd Flemisch
apl. Prof. Dr. rer. nat.Professor for Simulation Technology
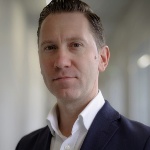
Sergey Oladyshkin
apl. Prof. Dr.-Ing.Project Leader, Research Project D03